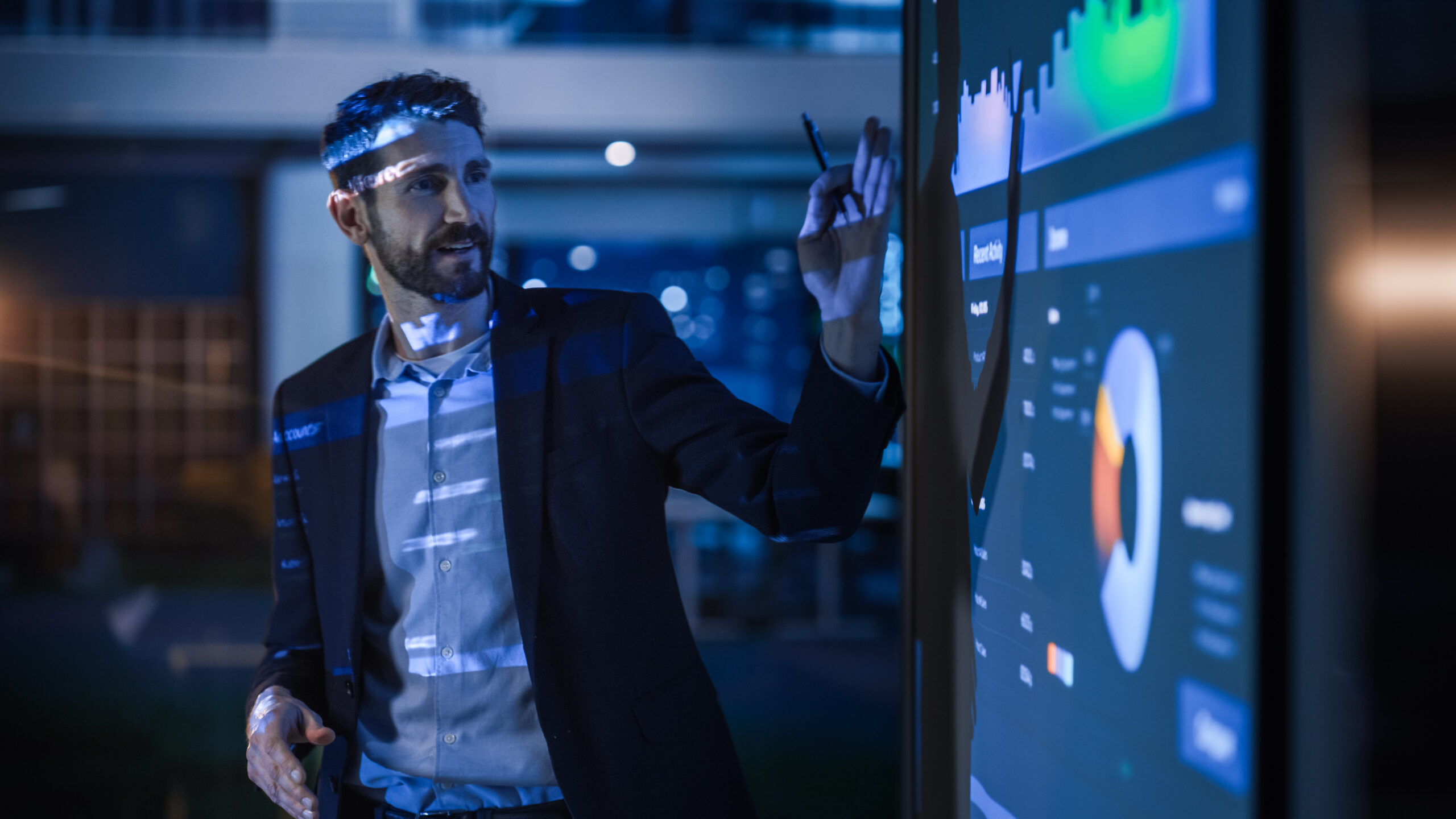
Key findings:
- A data lake serves as a central repository for comprehensive productivity insights.
- Data lakes support various data types, efficiently stores unstructured data and uses object storage for easy retrieval.
- Decision making is improved by uncovering patterns, improving decisions and enhancing productivity.
Your business might already be collecting data but without a central resource its full potential is untapped. So, it’s time to look at your options. Should that data be piped directly to a business intelligence program, or should it be mixed in a data lake or warehouse? The answer depends on your budget and what you’re looking to get out of your investment.
Centralising your data resource
If you’re just trying to automate a few reports and spend the bare minimum to make sure some dashboards are ready to be looked at every morning, a BI program is probably the solution. But for a more data-driven culture that sets a foundation for growth in a business, creating a central repository to marry things together is highly valuable, and for many organisations a data lake is the answer.
For the uninitiated, when we talk about data lakes, we’re referring to a central location that holds a large amount of data in a raw format. In a traditional database, data is arranged in a hierarchical or classified structure, in numbered tables and rows, for example. But a data lake has a flat architecture – that is, data is stored in a non-hierarchical manner.
A lake supports a wide range of data types, and efficiently stores unstructured or semi-structured data such as images, videos, and documents in a single, large repository. It does this through object storage, which labels data with metadata tags and a unique identifier, making it easier to locate and retrieve data, and improving performance.
Advantages of data lakes
Many executives recognise the value of pooling data. Take warehouse, logistics and transport, three areas of a business that might use a raft of different systems for employee timesheets, warehousing and Enterprise Resource Planning (ERP).
To track data across three different elements of a business individually is useful but bringing it into one central place allows for a more complete examination of productivity metrics.
Factoring in, for example, hours worked against volumes shifted and orders from your ERP creates rich data sets that ultimately make it easier for people across the business to identify inefficiencies, optimise routes, and enhance overall productivity.
There are many business leaders who are at the start of their data journey or have just been dabbling in data and haven’t yet recognised the need for external capability and support.
A classic data problem is linking a Customer Relationship Management (CRM) system into other systems. Often a business has a standalone CRM, which allows oversight of all interactions with various customers, but they can’t see when that actually translates into a transaction or actual benefit for the business.
A data lake is one possible mechanism for marrying customer and transaction data, which delivers better insights about how to move more customers from prospective to converted. Starting out in data does not have to be an all-or-nothing approach and indeed, it’s valuable to start with a small, well-defined project.
Client story: Data lake enhanced decision-making
Let’s take our client, PFD Foods Services as an example. This company was focused on developing a culture of safety, was already advanced in terms of data streaming from their trucks, and measured factors such as the duration of their runs, the routes taken and the types of deliveries.
All the data was there – so what would marrying it up bring to the table?
It allowed insights into what the drivers were reporting in terms of runs and deliveries, versus what the trucks were actually doing. Were the engines running while they were supposed to be taking a break? Were the drivers running the most efficient route?
This was a project viewed through a safety lens, not a tool for employee monitoring. They were not worried about catching people out but reinforcing a safety culture by making drivers accountable about taking breaks.
By creating a data lake and examining the full picture of the data, the company now had a better view of driver compliance regarding safety and an outlet for lifting productivity. Data lakes can be more cost-efficient in terms of storage and flexibility than data warehouses, which is another form of central repository, but one that works best when the data is structured. If you’re interested in learning more about PFD Food Services, read our client story here.
Governance is needed to maintain data quality
For businesses or organisations that rely on fast, real-time analytics of unstructured data, combined with analysis of more traditional structured data, the recent invention of ‘data lakehouses’ may be the most suitable structure. They can offer the best features of both data warehouses and data lakes by creating additional efficiencies and improved performance.
Whatever model is determined to be the best for your needs, don’t forget the governance piece. Ensure that robust frameworks are in place for data quality, security, and accessibility to ensure the data lake doesn’t become a swamp of poor-quality information, and ensure it has the necessary compliance with regulatory structures.
A data lake could transform how you use data, helping you quickly uncover patterns, improve decisions, and stay competitive. Marry up your data sources properly and you’ll have an excellent basis from which to extract rich data-driven insights and tackle complex problems.