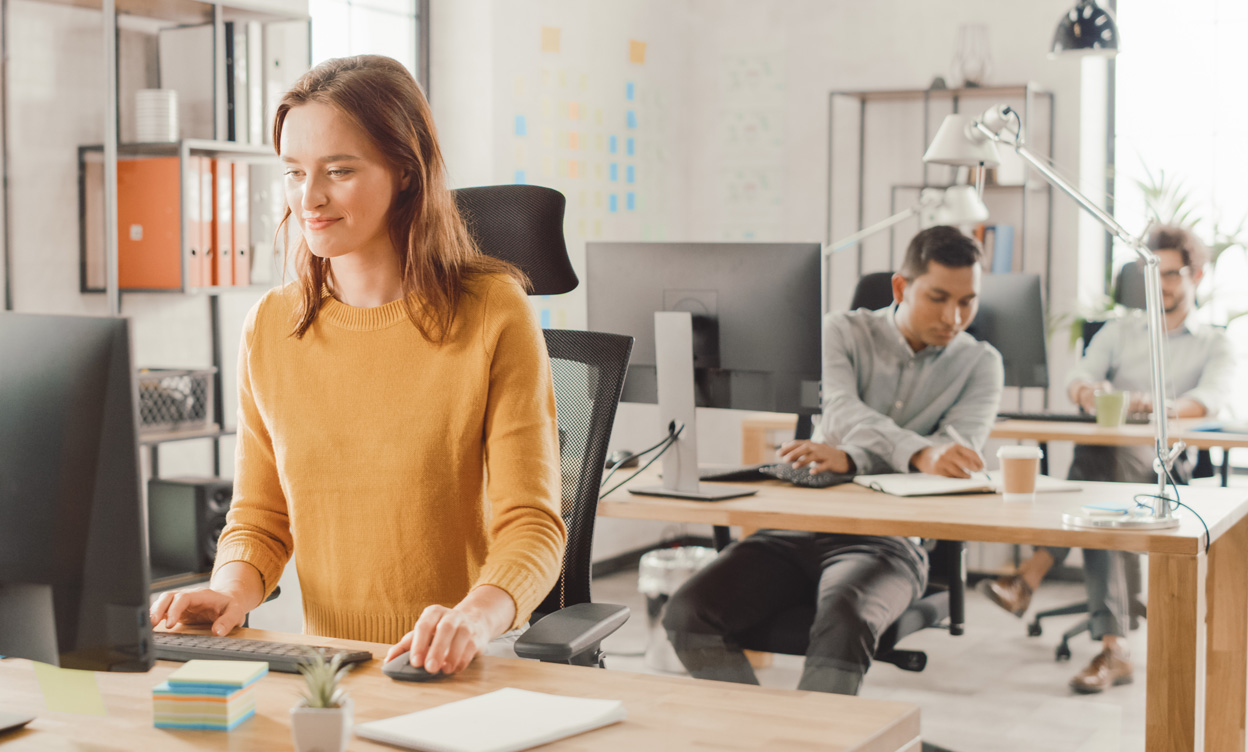
Unless you have been living under a rock, you will have heard of the implications for business of keeping untidy timesheets and payroll records — with increased calls for legal action against directors and employers who don’t comply.
New legislation recently introduced to the Parliament will allow stronger penalties, greater fines, and even jail time for employers found to have deliberately underpaid their workers, but this will come on top of already strong rules in many states designed to crack down on poor payment practices.
The Fair Work Commission reported at least seven cases of companies being fined in the first six months of the year in which payslips, timekeeping, and recordkeeping were found to be flawed, while Victoria’s Wage Theft Taskforce has taken action against multiple employers.
It’s a chilling thought for businesses that have traditionally relied on paper records.
Even with the best intentions — and the vast majority of businesses do their best to keep good records, navigate the complex awards, and ensure they are matching employees with their entitlements — systems can break down.
And when you are working with historic paper records, employers can be sorting through timesheets that are hard to read, poorly filled out, lack critical detail, or completed in barely legible scrawl.
But since employers must keep time and wages records for seven years, and those have to be readily accessible for any Fair Work Inspector and “legible”, there’s new interest in using machine learning to review, digitise and collate past employee records.
The good news is that computer vision and machine learning are improving all the time, making the process of reviewing, reading, recording, and flagging missing information easier.
The bad news? The records were kept by humans.
To understand the challenge a lot of businesses face when digitising reports, think about how you draw the number 7.
Does it look a little like a 1? Do you use a line slashed through the diagonal stroke on the 7 or hook your 1 at the top?
Or does your handwritten number 4 look like a 9? Your hurried 6 look a little like an 8?
A critical challenge for the digitising process is to review all the ways a human might scrawl the time they started or the number of hours they worked, as they race into the café or dash off their times after a long shift.
The technology to read loops and lines, and identify what is a digit and what is a random squiggle, is vastly improved, and we worked with Amazon Web Services on this process, leveraging their world-class computer vision technology to identify, classify, train and validate timesheet reading.
The second challenge, though, is the format in which that data has been kept. On one recent project, we reviewed over 50,000 handwritten timesheets that had been kept on more than 20 templates over several years.
Here, you are requiring the machine learning process to extract information from a particular column on one version of the timesheet document, only to have that same information in a different column on another style of page.
Again, there’s a process that you can undertake to help train the algorithm on multiple versions of documents, but this requires human feedback to keep the process moving.
A final challenge is the way those records have been kept.
Ideally, they would have been scanned with computer vision in mind, nice and straight, with the information clearly shown.
Of course, the people scanning get tired as well, and you can often find shadows, blurriness or folded pages that make the input less than ideal.
If they have been entered online at some point, it will have been a manual process, so errors can be replicated and compounded, dodgy maths applied or vital information missed so the paper record cannot easily be matched.
None of this should be a barrier for businesses looking to review and reconcile old records — in fact, it makes it more important that they do so.
But when you plan for the future, think about how any paper entries you keep today will cause you headaches down the line, when you need to deliver the next tranche of digital proof to regulators.